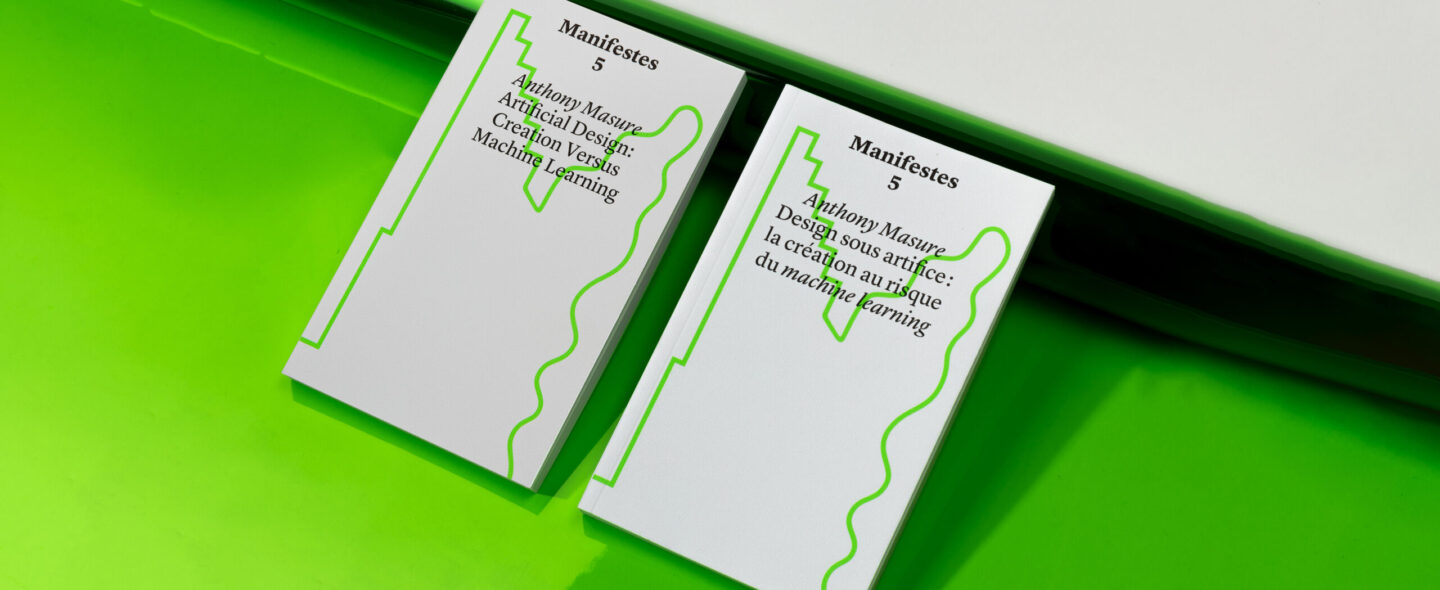
San Francisco – January 6, 2025
Designing with Machines: Rethinking Generative AI Beyond Replacement Narratives
First introduced to the general public through the launch of entertainment and creation-oriented services such as DALL-E (January 2021), Midjourney (July 2022), and ChatGPT (November 2022), machine learning technologies form the basis of what we now call “generative” artificial intelligence. These technologies enable the “generation” of digital objects—including text, images, sound, video, or 3D files—based on text prompts. These authorless productions are simultaneously a source of wonder and debate among the international creative community, which is alarmed by the threat these processes pose to the survival of design professions.
Overcoming the Man/Machine Polarization
The common media narrative, which focuses on the “replacement” of humans by machines, oversimplifies the conversation and undermines a more nuanced understanding of the social issues at stake. This framing distracts from the biases, limitations, and possibilities of these programs. A more relevant question is how machine learning technologies shape and redefine creation. Far from being reducible to facile slogans, these technologies challenge notions such as the ethos of designers, the nature of originality, and the definitions of aesthetic categories. The goal is not to glorify or demonize AI but to unpack the nuances and complexities of the theoretical, political, and practical issues it raises in design.
With this perspective in mind, a pilot project at the Geneva University of Art and Design (HEAD – Genève), titled “Design & Machine Learning” (2021–2023), explored these challenges. Funded by HES-SO and led by myself, the project addressed three key research questions:
- How can the automation inherent in AI technology be repurposed on a smaller scale, in a controlled and “tailor-made” way?
- How can machine learning be used to encourage invention and curiosity?
- How can design help defuse the dominant culture of AI?
The findings of this research, presented in the open-access essay Artificial Design: Creation Versus Machine Learning (HEAD – Publishing, 2023), propose new ways of thinking about AI and its implications in creative fields.
AI Beyond Human Intelligence
The first part of the book examines the origins of these technologies. It explains how the emergence of deep learning at the turn of the 2010s became the foundation for contemporary generative AI. Deep learning operates a paradigm shift: instead of humans writing computer programs, machines now write these programs from “data” (files) collected from the Web, which are converted into “vectors” (geometric coordinates). The result is a system that is structurally opaque—both in terms of its datasets and its processes. This opacity challenges conventional ethical and moral frameworks, as AI query results are produced based on statistical, not semantic, logic.
The book also highlights how contemporary AIs mimic human intelligence through a behaviorist approach, focusing solely on what can be verbalized toward an objective. This reflects a capitalist mindset that prioritizes productivity over exploration and dismisses approaches rooted in psychological dysfunctions, such as psychoanalysis.
Alternative ways of understanding intelligence could emerge by revisiting the cybernetics era (1948–1975), during which researchers explored multiple forms of intelligence, including animal cognition. For example, a doctoral thesis by Eloïse Vo at HEAD – Genève, in partnership with EPFL, revisits the history of the Dolphin House project. Funded by NASA in the 1960s, this initiative sought to communicate with dolphins in a custom-built house in the U.S. Virgin Islands. This historical detour reimagines AI from the perspective of synthesis rather than artificiality, shedding light on the relationship between human and non-human intelligence.
While works like the Atlas of AI (Kate Crawford, 2021) illuminate the darker economic, ideological, and ecological dimensions of AI production, there remains a need for a “counter-atlas” that explores alternative genealogies and practices of AI. Such a project would focus on the situated experiences of actors, imaginaries, and other heterotopias.
Towards a Balkanization of the Web
The second part of Artificial Design delves into the risks and biases associated with AI. While these risks may initially seem unrelated to creative professions, they are deeply connected to questions of responsibility (social purpose) and the construction of alternatives (resisting dominant industrial practices).
Since the early 2020s, generative AI has transitioned from a science-fiction concept to an integral part of everyday life. As Rebecca Coleman notes, this shift occurs when “the technologies that offer fantasy conform to the needs of everyday life.” At this juncture, AI must be re-imagined.
A key distinction lies in AI’s profoundly political character, which generates significant social risks and challenges. Commercial generative AI, driven by an alliance between technology and ultra-liberalism, undermines the foundations of Western democracies. It challenges notions of truth, privacy, property, and shared values. Moreover, AI accelerates the decline of the Web, which has been under threat since the late 2000s due to smartphone app stores. The Web is now fragmented into geographical blocks (the West, China, Iran, Russia, etc.), creating a “splinternet” that dismantles Tim Berners-Lee’s vision of global knowledge sharing.
From Emancipation to Automation
By relying on data from the public Web—data that is unrepresentative of the world’s diversity—generative AI reproduces and reinforces existing power dynamics. This enables those who control these technologies to dominate while further marginalizing vulnerable populations. The labor required to sustain these systems is often outsourced to the Global South (e.g., Kenya, Vietnam, Venezuela), where workers are described as “click proletarians” by sociologist Antonio Casilli (Waiting for Robots, 2019).
These technologies also compromise privacy, collecting and exploiting personal data for commercial or surveillance purposes. Legally, generative AI (even open source ones) often relies on datasets collected without authorization, raising significant copyright concerns. Some artists feel dispossessed of their work, and legal battles are underway against companies profiting from these productions.
Historically, the promise of emancipation through technology has been replaced by automation—or worse, by the demand for adaptation (to be trained or replaced). Despite its successes, generative AI has yet to be leveraged for social projects that promote widespread emancipation or civic consciousness. Addressing these challenges requires reimagining AI through diverse histories and counter-histories.
Mind Over Matter
The third and final part of Artificial Design explores the new forms of production enabled by AI. The promise of automation, which reduces invention to the reproduction of the past (e.g., an author’s style), is tied to profit-making. It should not be mistaken for the foundation of art and design, which lies in exploring the potential of a technique to uncover alternative ways of creating beyond those dictated by the economic system.
Discourses promoting generative AI often frame it as a neutral “tool” or intermediary shaped by human intentions. This perspective reinforces the essentialist human/machine dichotomy, paradoxically stifling genuine innovation and ignoring anthropology. Instead, art and design offer opportunities for discovery and surprise, rooted in material engagement and non-verbal aesthetic experience.
To counter the standardization of aesthetic production, designers must engage deeply with techniques, embracing their potential to deviate from prescribed norms. Only through such engagement can we resist the reductive logic of generative AI and its focus on efficiency at the expense of creativity.
Pluralizing AI
To explore alternatives to imitation and standardization, our essay includes contributions from European design studios working prospectively with AI. These interviews, conducted by teacher and designer Alexia Mathieu in 2021–2022 (at the start of consumer AI), outline five creative potentialities:
- Revealing the dynamics of standardization: Highlighting what is already formatted in our daily lives.
- Empowering the injunction to simplicity: Emphasizing the human and material dimensions of technologies.
- Playing with the hazards and limits of prediction: Exploring deviations rather than alignment with intent.
- Translating cultural codes: Transposing concepts across mediums (e.g., images into sound, 3D, video, etc.).
- Inventing new modes of collaboration: Treating the machine as a partner, not an assistant.
One example is the Made in Machina/e project (2018) by studio OIO (Simone Rebaudengo and Sami Niemelä). By hybridizing datasets from two opposing cultures—Chinese electronic components and Northern European product design—the project creates unexpected objects like a smartphone chandelier or a glass-ceramic video game controller. This approach demonstrates how technologies cannot be reduced to a one-way street: AI can be pluralized by engaging with it critically and creatively.
Generative AI: A Starting Point, Not an End
Generative AI, like all technologies, must be understood as a starting point rather than an endpoint.
By focusing on what already exists, instead of leaping into utopian visions, we can navigate these technologies critically, creatively, and ethically.
Following the publication of my essay Artificial Design in March 2023, new research projects have emerged that I am currently developing or supporting at HEAD – Genève. These projects aim to test and refine the five “creative potentialities” discussed earlier, allowing for their evolution and redefinition.
- First and foremost, the concept of an “intelligence of the artificial” highlights the importance of embracing diverse forms of intelligence—not purely human or machine-based, but those existing in the in-between spaces. Exploring these liminal areas opens up opportunities for new types of collaboration between living beings and machines. This task becomes even more urgent with the rise of so-called “neural interfaces,” which once again promise a direct connection between the human brain and machines.
- In addition, the recent commercial development of “sexbots” and “AI Girlfriends” warrants attention. These objects and services, which model sexual and emotional norms via AI, need to be reexamined and reinvested in from feminist, queer, and anti-ableist perspectives. A related research project, “Fucking Tech!” (2024–2027), led by myself and Saul Pandelakis, seeks to address these issues critically.
- Another avenue is to revisit the notions of the false and the original (Pierre Leguillon, 2023-2025), which are constantly shifting across time and space. This approach could shed new light on the current challenges of AI disinformation. Could we not reactivate the long tradition of simulation—or even hoaxes—to disrupt conventional design practices and question its dominant figures and rituals?
- A particularly promising and actionable direction is the creation of small data sets crafted by artists and designers (Alexia Mathieu, Datacraft, 2023-2025). This localized, “tailor-made” approach distances practitioners from the opacity and normative weight of large language models. By controlling a greater portion of the work process, these smaller datasets position AI as a step within the creative process rather than an endpoint.
Conclusion: In praise of deceptive technologies
Returning to the 3 key questions raised earlier, we can now propose some pathways for exploration:
- How can the automation inherent in technology be repurposed on a smaller scale, in a controlled and “tailor-made” way?
Given the ethical and environmental challenges of building large datasets and the computing power required to process them, fragmenting these datasets into smaller, localized sets is a fruitful strategy. This not only allows for greater control over the process but also has the potential to produce more precise and contextually relevant results (an all-purpose model may be “good” everywhere but excellent nowhere). - How can machine learning be used to encourage invention and curiosity?
We must move away from the paradigm of the “magic button” that delivers immediate, effortless results. Instead, we should invest time in understanding the theoretical and technical foundations of AI. This could mean creating specific works where AI is mobilized only at certain stages of the creative process. - How can design help defuse the dominant culture of AI?
Historically, design has carried the utopian ideal of precision and accuracy in industrial production. This legacy positions design as a potential counterforce, capable of exposing the problematic conditions under which commercial generative AI is currently deployed.
Emerging technologies like AI are frequently accompanied by promises of profitability or human enhancement. To counter these claims, we propose embracing the idea of deceptive technologies—and seeing this deception as a positive force.
In his essay Décevoir est un plaisir (Disappointment is a Pleasure, 2024), philosopher Laurent de Sutter distinguishes between projection (casting our hopes into a distant future) and orientation (focusing on where we are and what we have). The latter, he argues, allows us to move forward step by step, exploring the unknown and adapting along the way. In contrast, projection assumes a great leap forward that resolves everything in one stroke, leaving no room for discovery. While this attitude avoids the disappointment of unmet expectations, it risks missing what is already present, freezing the possibility of action in the here and now.
Laurent de Sutter’s distinction between “projection” (looking toward a distant future) and “orientation” (focusing on the key elements of our current situation) emphasizes the value of making the most of what is already available to us: “Any orientation is only ever a starting point – whereas a projection presupposes that we have already arrived before the first step has been taken […]. In orientation, we only progress step by step, stage by stage, in the exploration of what was unknown and which, from becoming known, only gives way to another unknown; in projection, we are content to make one great leap, which settles everything (p. 25-26).”
Applied to the fields of generative AI and design, these reflections underscore two key points:
- The absurdity of understanding creation as the slavish reproduction of the past, where nothing exceeds the boundaries of the known.
- The danger of expecting technologies to spare us the effort of doing, with a mystical belief that they will resolve our existential challenges.
As technologies promise greater automation, they simultaneously distance us from the possibility of emancipation. The idea of working “with” techniques has been sidelined; instead of being approached as materials to engage with, technologies are presented as horizons of expectation.
To counter this trend, it would be better to think of design as an art of orientation: working creatively with what is already present and resisting the temptation to envision an “end of time” where all problems are solved by technological artifice.
About the author
-
Bio
Anthony Masure
Associate Professor and Dean of Research, Geneva University of Art and Design (HEAD – Genève, HES-SO)As a practice-based tech critic, he aims to understand and shape how emerging technologies can craft a more sustainable future through design. His research is currently focused upon artificial intelligence, blockchain and gaming. He was co-curator of the exhibition “Assembling Intelligence: Hybrid Strategies for AI, Art, and Design” (Swissnex San Francisco, August to November 2024). He is the author of the essays Design and Digital Humanities (2017) and Artificial Design: Creation Versus Machine Learning (2023). He is also a co-founder of the research journal Back Office.